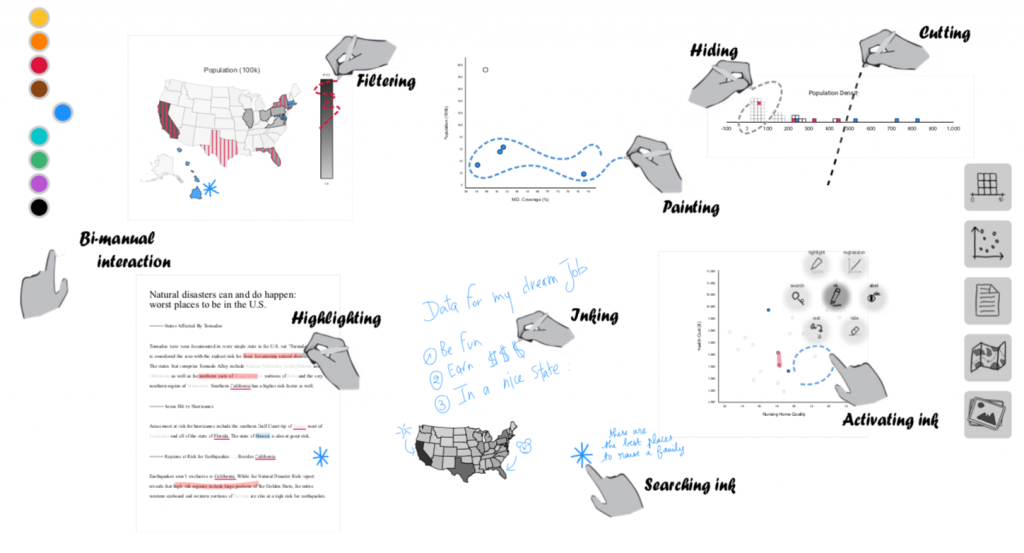
Contributors:
Hugo Romat, Nathalie Henry Riche, Ken Hinckley, Bongshin Lee, Caroline Appert, Emmanuel Pietriga, and Christopher Collins
During sensemaking, people annotate insights: underlining sentences in a document or circling regions on a map. They jot down their hypotheses: drawing correlation lines on scatterplots or creating personal legends to track patterns. We present ActiveInk, a system enabling people to seamlessly transition between exploring data and externalizing their thoughts using pen and touch. ActiveInk enables the natural use of a pen for active reading behaviours while supporting analytic actions by activating any of these ink strokes. Through a qualitative study with eight participants, we contribute observations of active reading behaviours during data exploration and design principles to support sensemaking.
This research was given an honourable mention at CHI 2019.
Learn more about ActiveInk by visiting the project’s website and be sure to check out Microsoft’s blog post on ActiveInk.
Publications
-
[pods name="publication" id="4209" template="Publication Template (list item)" shortcodes=1]