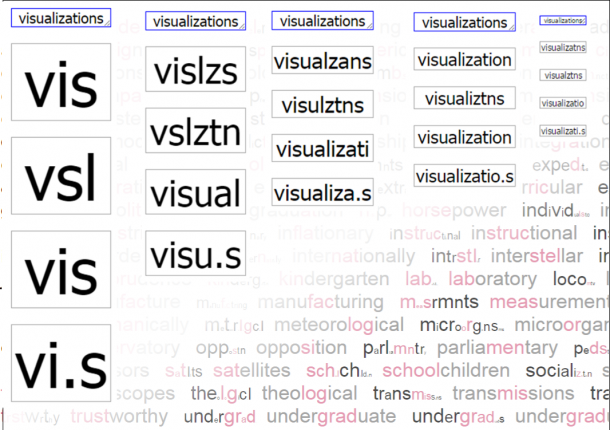
Contributors:
A known problem in information visualization labelling is when the text is too long to fit in the label space. There are some commonly known techniques used in order to solve this problem like setting a very small font size. On the other hand, sometimes the font size is so small that the text can be difficult to read. Wrapping sentences, dropping letters and text truncation can also be used. However, there is no research on how these techniques affect the legibility and readability of the visualization. In other words, we don’t know whether or not applying these techniques is the best way to tackle this issue. This thesis describes the design and implementation of a crowdsourced study that uses a recommendation system to narrow down abbreviations created by participants allowing us to efficiently collect and test the data in the same session. The study design also aims to investigate the effect of semantic context on the abbreviation that the participants create and the ability to decode them. Finally, based on the study data analysis we present a new technique to automatically make words as short as they need to be to maintain text legibility and readability.
Based on this project we implemented and made available online an API that allows other programmers to use our abbreviation algorithm in their web applications.
Check out our GitHub Repository for source code related to this project.
Download the crowd-sourced dataset.
For some demos applying our “Abbreviation on Demand” algorithm, and some visualizations of our study data access: http://vialab.science.uoit.ca/abbrVisualization/
Publications
-
[pods name="publication" id="4248" template="Publication Template (list item)" shortcodes=1]
[pods name="publication" id="4251" template="Publication Template (list item)" shortcodes=1]
[pods name="publication" id="4254" template="Publication Template (list item)" shortcodes=1]