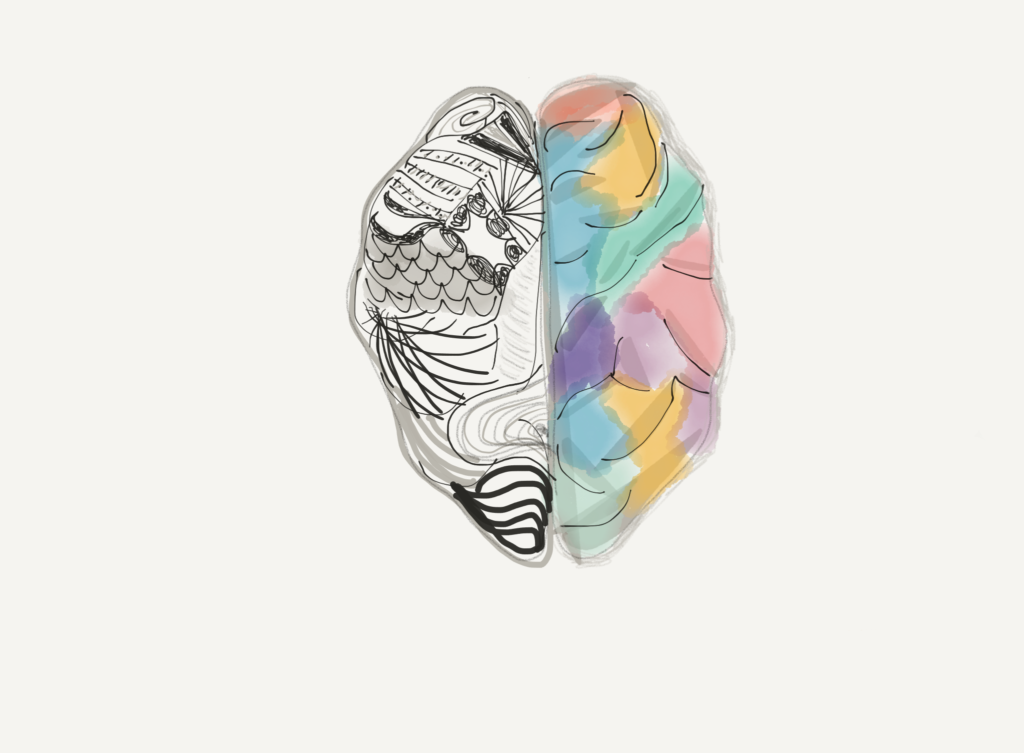
Contributors:
First language (L1) influence errors are very frequent in English learners (L2), even more so when the learner’s proficiency level is higher (upper-intermediate/advanced). Our project aims to analyze errors made by learners from specific L1’s using learner corpora. Based on the analysis we want to focus on a specific type of error and research a way to identify it automatically in learners’ essays depending on their L1. This would allow us to implement an application that helps English as Second Language (ESL) students to identify and analyze their errors and to better understand the reasoning behind them, consequently improving the students’ English level.
About the EduApps initiative
EduApps is a suite of apps housed in an online environment that focuses on the health, well-being and development of one’s mind, body and community. Our research project titled, “There’s an App for That” is investigating the design process, development, implementation and evaluation of this suite of educational apps. Specifically, we are interested in helping students build confidence and competence in the cognitive, socio-emotional and physical domains. We are also interested in the impact a learning portal can have on students’ learning, teachers and the surrounding community. We hope that our research can build capacity for investigating and affecting innovation in formal and informal education settings in the use of digital technology. We have partnered with school boards and community organizations to develop and research the apps. More about each of the domains — their purpose, apps and related research can be found at http://eduapps.ca/.
Publications
-
[pods name="publication" id="4191" template="Publication Template (list item)" shortcodes=1]