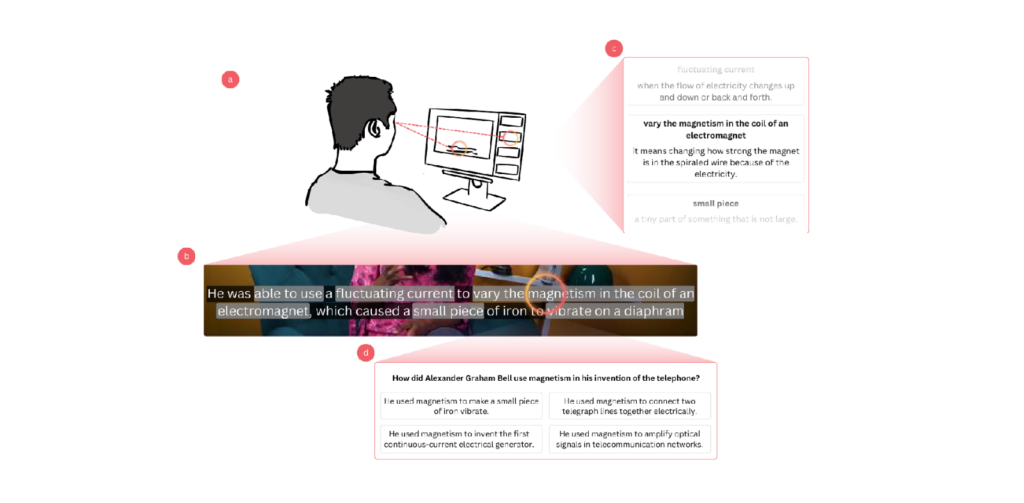
Contributors:
Benedict Leung, Mariana Shimabukuro, Matthew Chan, Christopher Collins
Abstract
Effective comprehension is essential for learning and understanding new material. However, human-generated questions often fail to cater to individual learners’ needs and interests. We propose a novel approach that leverages a gaze-driven interest model and a Large Language Model (LLM) to generate personalized comprehension questions automatically for short (∼10 min) educational video content. Our interest model scores each word in a subtitle. The top-scoring words are then used to generate questions using an LLM. Additionally, our system provides marginal help by offering phrase definitions (glosses) in subtitles, further facilitating learning. These methods are integrated into a prototype system, GazeQ-GPT, automatically focusing learning material on specific content that interests or challenges them, promoting more personalized learning. A user study (𝑁 = 40) shows that GazeQ-GPT prioritizes words in the fixated gloss and rewatched subtitles with higher ratings toward glossed videos. Compared to ChatGPT, GazeQ-GPT achieves higher question diversity while maintaining quality, indicating its potential to improve personalized learning experiences through dynamic content adaptation.
GazeQ-GPT Video Figure
Publications
-
[pods name="publication" id="9529" template="Publication Template (list item)" shortcodes=1]